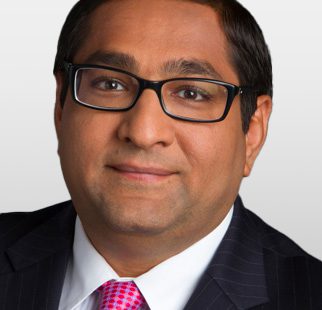
Ash M. Patel
Chief Technology and Transformation Officer
In the rapidly evolving AI field, particularly within the CPG and retail industries, the issue of “hallucinations” in AI outputs has become a pressing concern. Under intense market competition, many companies have felt the pressure to prioritize speed over quality, often at the expense of quality control, robust data inputs, and adequate training time.
Hallucinations — instances where AI generates false or misleading information — can arise from several factors:
- Lack of domain knowledge: The specific knowledge related to a particular business process or business context is not used in the training corpus for the model to correctly answer the question.
- Data quality: Models can be challenged by having data that is too stale or incomplete for accurate answers to be generated.
- Inability to answer the question: Generative AI models will attempt to answer questions that are better answered by traditional AI or algorithms, producing results that are incorrect or don’t make sense.
A training corpus is a collection of digital assets and associated metadata that is used to train a machine learning model. This is an essential part of AI automation.
The rush to launch AI solutions often means insufficient time is devoted to training models properly, exacerbating the issue. We resisted this panic when developing Liquid AI™, and took over five years to develop and tune the solution before launching it to clients.
The risks associated with AI hallucinations are far from negligible. They can lead to the spread of misinformation within an organization, poor decision-making based on inaccurate data, and ultimately, a loss of trust in AI solutions. This is particularly concerning in industries where decisions have significant financial and strategic implications.
To combat these challenges, it’s imperative to prioritize quality over speed. This entails investing in:
- Data integrity: Ensure the data used to train AI models is of high quality and is representative.
- Comprehensive training: Allocate sufficient time and resources to thoroughly train AI models.
- Quality assurance: Implement stringent testing and validation processes to identify and rectify hallucinations before they affect users.
In my view, success comes from focusing on a long-term commitment to developing AI solutions that are not just innovative – they’re also reliable and trustworthy.
This is something we take seriously at Circana. Our market and consumer data is crucial to the value of Liquid AI due to its breadth, depth, and quality. With a proprietary directory that includes more than 225 million items and more than 1 million richly coded attribute values, we provide detailed, nuanced insights essential for accurate analysis and decision-making.
Because we calibrate point-of-sale (POS) data with more than 2,000 retail partners across more than 14.5 million stores means our insights reflect market reality and we can maintain accuracy in reporting consumer drivers of industry trends.
This extensive data network ensures that Liquid AI’s insights are grounded in a complete picture of the consumer goods market. This makes it a trusted tool for businesses navigating the complex landscape of the CPG and general merchandise industries.
In addition to ensuring quality data inputs, my advice to teams considering their next steps into AI would be to consider various strategies to ensure outputs can be relied upon for business decisions:
- Robust training: Ensure a diverse and high-quality dataset for training the AI to reduce the likelihood of hallucinations.
- Continuous monitoring: Regularly evaluate the AI’s performance and the accuracy of its outputs to help catch hallucinations early.
- User education: Train users to recognize potential AI hallucinations and verify critical information through additional sources.
- Feedback loops: Implement mechanisms for users to report inaccuracies to help improve the AI model over time.
It’s also crucial to incorporate traditional or predictive AI with generative AI. This integration ensures the right tool is used for the right question, leveraging domain knowledge to determine the most appropriate AI approach. By combining the strengths of different AI methodologies, businesses can achieve more accurate and reliable outcomes, enhancing their decision-making processes and overall efficiency.
In conclusion, while AI hallucinations present a hurdle in the use of AI-based solutions, with careful management and a proactive approach, these issues can be mitigated. The key is to maintain a balance between leveraging the powerful capabilities of AI and ensuring the reliability of the insights it provides. That’s how to ensure AI continues to be a valuable tool for driving business growth and providing actionable insights, rather than a source of potential misinformation and risk.
Get insights straight to your inbox